CRM Trends to Watch: How Machine Learning and Big Data Are Shaping the Future – that’s the burning question on every forward-thinking business leader’s mind. Forget dusty spreadsheets and manual data entry; the future of customer relationship management is here, and it’s powered by AI and the sheer volume of data we’re generating daily. This isn’t just about automating tasks; it’s about understanding your customers on a deeper level, predicting their needs, and delivering personalized experiences that build loyalty and drive growth. Get ready to dive into the exciting world of smart CRM.
This deep dive explores the evolution of CRM, from its traditional roots to its current AI-powered iteration. We’ll unpack how machine learning is revolutionizing lead scoring, predictive analytics, and customer segmentation, showcasing real-world examples of how businesses are using these advancements to gain a competitive edge. We’ll also tackle the challenges, from data security to integration complexities, and paint a picture of what the future holds for CRM in an increasingly data-driven landscape.
The Evolving Role of CRM in a Data-Driven World
The world of Customer Relationship Management (CRM) is undergoing a dramatic transformation, shifting from a simple contact database to a sophisticated, AI-powered engine driving business growth. This evolution is fueled by the exponential growth of data and the increasing sophistication of machine learning algorithms, fundamentally altering how businesses understand and interact with their customers. We’re moving beyond basic contact management into a realm of predictive analytics and hyper-personalization, all powered by the insights gleaned from big data.
This shift from traditional CRM to AI-powered systems represents a quantum leap in capabilities. Traditional CRM systems, while useful for organizing customer information, largely relied on manual processes and lacked the predictive power to anticipate customer needs. AI-powered systems, however, leverage machine learning to analyze vast datasets, identifying patterns and trends that would be impossible for humans to discern. This allows for more proactive engagement, personalized experiences, and ultimately, improved customer satisfaction and loyalty.
Machine learning and big data are revolutionizing CRM, offering hyper-personalized experiences and predictive analytics. To truly leverage these advancements, businesses need to adopt smart strategies, and that’s where understanding best practices becomes crucial. Check out this insightful guide on 5 CRM Best Practices Every Business Should Adopt in 2025 to ensure you’re maximizing the potential of these emerging technologies.
Ultimately, integrating these best practices will unlock the full power of data-driven CRM in the future.
Big Data’s Impact on CRM Functionality and Customer Insights
Big data is the lifeblood of modern CRM. The sheer volume, variety, velocity, and veracity of data available today – from website interactions and social media activity to purchase history and customer service interactions – provides an unparalleled opportunity to understand customer behavior at a granular level. This data, when properly analyzed, reveals valuable insights into customer preferences, purchase patterns, and even potential churn risk. For example, analyzing social media sentiment can provide early warnings of potential customer dissatisfaction, allowing businesses to address issues proactively. Similarly, analyzing purchase history can reveal cross-selling and upselling opportunities, boosting revenue and customer lifetime value.
Real-Time Data Processing and Enhanced CRM Effectiveness
Real-time data processing is crucial for maximizing the effectiveness of AI-driven CRM. The ability to analyze and respond to customer interactions instantaneously allows for immediate personalization and improved customer service. Imagine a scenario where a customer is browsing your website and adding items to their cart but abandoning it. A real-time CRM system can trigger a targeted email offering a discount or free shipping, potentially converting a lost sale into a successful one. This immediate responsiveness is a key differentiator between traditional and modern CRM systems, enabling businesses to engage with customers in a more meaningful and timely manner.
Comparison of Traditional CRM and AI-Driven CRM Features
Feature | Traditional CRM | AI-Driven CRM |
---|---|---|
Data Analysis | Manual, limited insights | Automated, predictive analytics, real-time insights |
Customer Segmentation | Basic demographic segmentation | Sophisticated behavioral segmentation, personalized targeting |
Customer Interaction | Reactive, primarily transactional | Proactive, personalized engagement, predictive support |
Predictive Capabilities | Limited or absent | Churn prediction, sales forecasting, opportunity identification |
Machine Learning Applications in CRM
Machine learning is no longer a futuristic concept; it’s actively reshaping how businesses interact with their customers. By leveraging the power of algorithms and vast datasets, companies are gaining unprecedented insights into customer behavior, leading to more effective strategies and ultimately, increased profitability. This section explores three key areas where machine learning significantly boosts CRM performance.
Predictive Analytics for Customer Segmentation and Targeting
Predictive analytics, powered by machine learning, allows businesses to move beyond basic demographic segmentation. Instead of relying on broad strokes, companies can now create highly granular customer segments based on predicted behavior, lifetime value, and propensity to churn. This level of detail allows for hyper-personalized marketing campaigns and product recommendations, significantly increasing engagement and conversion rates. For example, a clothing retailer might use predictive analytics to identify customers likely to purchase winter coats within the next month, enabling targeted email campaigns showcasing new arrivals and special offers. This precision targeting reduces wasted marketing spend and maximizes ROI.
Lead Scoring and Prioritization with Machine Learning
Effective lead management is crucial for sales success. Machine learning algorithms can analyze vast amounts of data – from website activity to social media interactions – to assign scores to leads based on their likelihood of conversion. This automated lead scoring process helps sales teams prioritize their efforts, focusing on high-potential leads and freeing up time to nurture those with lower scores. Imagine a SaaS company using machine learning to identify leads who have downloaded multiple white papers, attended webinars, and visited pricing pages. These leads would receive a higher score, indicating a stronger likelihood of conversion, and would be prioritized for contact by the sales team.
Enhanced Customer Service Through Machine Learning
Machine learning is revolutionizing customer service by automating tasks and providing personalized support. Chatbots powered by machine learning can handle routine inquiries, freeing up human agents to focus on more complex issues. Furthermore, machine learning algorithms can analyze customer interactions to identify patterns and predict potential problems, allowing businesses to proactively address customer concerns and prevent negative experiences. For example, a telecommunications company might use machine learning to identify customers who are experiencing frequent service interruptions, enabling proactive outreach to minimize frustration and churn.
Lead Scoring Process Flowchart
The process of lead scoring using machine learning can be visualized in a flowchart:
[Imagine a flowchart here. The flowchart would begin with “Lead Data Input” (website activity, email engagement, social media interactions, etc.). This would feed into a “Machine Learning Model” box. The model would process the data and output a “Lead Score.” This score would then be used for “Lead Prioritization” (high-score leads prioritized for sales contact, low-score leads nurtured through marketing). Finally, there would be an output of “Sales and Marketing Actions” based on the lead score.] The flowchart visually represents how raw data is transformed into actionable insights, guiding sales and marketing efforts for optimal results. The accuracy and effectiveness of the lead scoring depend heavily on the quality and quantity of the data fed into the machine learning model, as well as the sophistication of the algorithm itself.
Big Data Analytics for Enhanced Customer Understanding
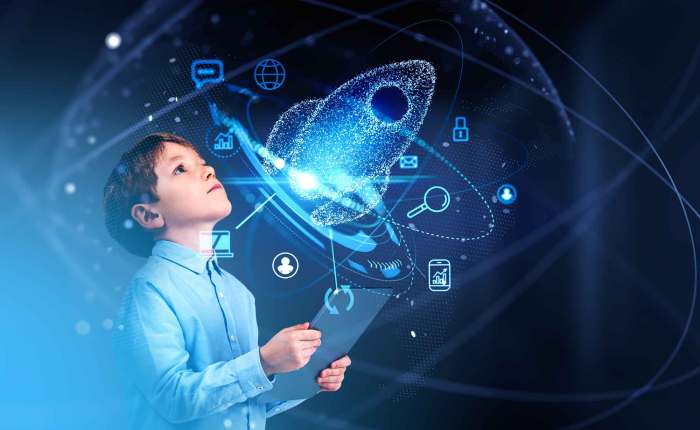
Source: kleinezeitung.at
In today’s hyper-connected world, businesses swim in a sea of data. Understanding this data – the likes, the clicks, the purchases, the service calls – is no longer a luxury; it’s a necessity for survival. Big data analytics provides the life raft, allowing companies to navigate this ocean and glean invaluable insights into customer behavior, preferences, and needs. This leads to more effective marketing, improved customer service, and ultimately, a stronger bottom line.
Big data analytics helps decipher complex customer behavior patterns by identifying trends and correlations that would be impossible to spot with traditional methods. Imagine trying to manually analyze millions of customer interactions to find a common thread. Big data analytics uses sophisticated algorithms to sift through this massive amount of information, revealing hidden patterns in purchasing habits, website navigation, social media engagement, and more. For example, analyzing website traffic data can reveal which pages are most popular, which products are frequently viewed together, and which marketing campaigns are driving the most conversions. This level of granular detail provides a much clearer picture of the customer journey than ever before.
Personalized Marketing Through Big Data
CRM systems leverage big data to create highly personalized marketing campaigns. By analyzing customer data – demographics, purchase history, website activity, social media interactions – CRMs can segment customers into highly specific groups with similar characteristics and needs. This allows businesses to tailor their messaging, offers, and product recommendations to resonate more deeply with each segment. For instance, a clothing retailer might use big data to identify customers who frequently purchase athletic wear and then target them with ads for new running shoes or fitness apparel. This targeted approach leads to higher conversion rates and increased customer loyalty. A well-executed personalized email campaign, for example, could significantly boost sales compared to a generic blast email.
Improving Customer Service and Support with Big Data
Big data also revolutionizes customer service and support. Analyzing customer service interactions – emails, calls, chat logs – can identify common issues, pain points, and areas for improvement. For example, a surge in complaints about a particular product feature might indicate a design flaw or a need for improved documentation. Predictive analytics, a subset of big data analytics, can even forecast potential customer service issues before they arise. By analyzing historical data, a CRM system might predict a spike in support tickets related to a specific product during a particular season, allowing the company to proactively increase staffing levels and prepare for the increased demand. This proactive approach minimizes customer frustration and improves overall satisfaction.
Actionable Insights from Customer Data Analysis
The power of big data analytics lies in its ability to deliver actionable insights. Analyzing customer data can reveal a wealth of information that directly impacts business decisions. Here are some examples:
- Identify high-value customers: Pinpoint your most loyal and profitable customers to focus your efforts on retaining them.
- Predict customer churn: Identify customers at risk of churning and proactively intervene with targeted offers or support.
- Optimize pricing strategies: Understand price sensitivity and adjust pricing to maximize revenue.
- Improve product development: Gather feedback and insights to inform the development of new products and features that meet customer needs.
- Personalize the customer experience: Tailor communications and offers to individual customers based on their preferences and behavior.
Improving Customer Experience through AI and Big Data
The marriage of AI and big data is revolutionizing customer experience, moving beyond simple interactions to proactive, personalized service. By leveraging the insights gleaned from massive datasets and the analytical power of AI, businesses are crafting customer journeys that are not only smoother but also deeply satisfying. This allows for a more efficient and effective use of resources, ultimately leading to increased customer loyalty and higher profitability.
AI-powered tools are no longer a futuristic fantasy; they’re actively shaping how businesses interact with their customers, leading to a more personalized and efficient experience for everyone involved. The ability to anticipate customer needs and proactively address potential issues is a game-changer in today’s competitive landscape.
AI-Powered Chatbots Enhance Customer Engagement and Support
AI-powered chatbots are transforming customer service by providing instant, 24/7 support. These bots can handle routine inquiries, freeing up human agents to focus on more complex issues. Furthermore, advanced chatbots can learn from past interactions, improving their ability to understand and respond to customer needs over time. For instance, a chatbot for an e-commerce site can instantly answer questions about shipping times, track orders, and even process returns, all without human intervention. This immediate response time significantly boosts customer satisfaction and reduces wait times, leading to a more positive overall experience. The integration of natural language processing (NLP) allows these chatbots to understand and respond to customer queries in a natural and conversational way, making the interaction feel more human.
Predictive Modeling Anticipates Customer Needs
Predictive modeling, fueled by big data analytics, allows businesses to anticipate customer needs and proactively address potential problems. By analyzing past customer behavior, purchase history, and other relevant data, businesses can identify patterns and predict future actions. For example, a telecommunications company might predict which customers are likely to churn based on their usage patterns and customer service interactions. This allows the company to proactively reach out to these customers with retention offers, potentially preventing churn and retaining valuable business. This proactive approach transforms the customer experience from reactive to preventative, fostering loyalty and strengthening the customer relationship.
Sentiment Analysis Improves Customer Satisfaction
Sentiment analysis uses AI to gauge customer opinions from various sources, such as social media, surveys, and customer reviews. By analyzing the emotional tone of this data, businesses can identify areas for improvement and address negative feedback promptly. For example, a negative sentiment surrounding a particular product feature can be identified and addressed through product updates or improved customer support. This allows companies to react quickly to customer concerns and demonstrate a commitment to continuous improvement. The insights gained from sentiment analysis are invaluable for shaping future strategies and enhancing customer satisfaction.
AI Improves Customer Experience: A Scenario
Imagine Sarah, a busy professional, needs to replace her broken phone. She visits an online retailer’s website. An AI-powered chatbot immediately greets her, offering assistance. Sarah explains her situation. The chatbot, using predictive modeling based on her past purchases and browsing history, suggests several phone models tailored to her needs and budget. It also proactively offers expedited shipping to minimize disruption. Later, after receiving the phone, Sarah posts a positive review on social media. The retailer’s sentiment analysis system flags this positive feedback, further reinforcing the effectiveness of its AI-driven customer service strategy. The entire experience, from initial problem to resolution, is seamless and efficient, leaving Sarah feeling valued and satisfied.
Challenges and Opportunities in Integrating AI and Big Data with CRM
Integrating AI and big data into CRM systems promises a revolution in customer relationship management, offering unprecedented insights and automation capabilities. However, this transformation isn’t without its hurdles. Successfully navigating these challenges unlocks significant opportunities for businesses to gain a competitive edge in today’s data-driven landscape.
Data Security and Privacy Concerns
The increasing reliance on AI and big data in CRM necessitates robust data security measures. Storing and processing vast amounts of sensitive customer information, including personal details, purchase history, and preferences, creates a significant vulnerability to data breaches and misuse. Compliance with regulations like GDPR and CCPA is paramount, requiring organizations to implement stringent security protocols, data encryption techniques, and access control mechanisms. Failure to adequately protect customer data can lead to severe financial penalties, reputational damage, and loss of customer trust – a far more costly outcome than investing in robust security infrastructure upfront. Consider the case of Equifax in 2017, where a data breach exposed the personal information of millions of consumers, resulting in significant financial losses and lasting reputational harm.
Data Integration and Interoperability Challenges
Many businesses operate with disparate CRM systems, legacy applications, and data silos. Integrating AI and big data solutions effectively requires seamless data flow across these systems. Lack of interoperability creates inconsistencies, data duplication, and difficulties in generating a holistic view of the customer. This fragmented data landscape hinders the ability of AI algorithms to accurately analyze customer behavior and predict future actions. For example, a company might have customer data spread across its marketing automation platform, e-commerce website, and point-of-sale systems. Without proper integration, the AI system will struggle to create a unified customer profile, limiting its effectiveness in personalized marketing campaigns. Effective data integration requires careful planning, the adoption of standardized data formats, and the use of robust data integration tools.
Overcoming Integration Challenges: Strategies and Best Practices
Successfully integrating AI and big data into CRM requires a multi-faceted approach. This involves not only technological solutions but also strategic planning, change management, and a commitment to data governance. Organizations should prioritize a phased implementation approach, starting with a pilot project to test the feasibility and effectiveness of the chosen solutions. This iterative process allows for continuous improvement and minimizes the risk of large-scale failures. Furthermore, investing in skilled data scientists and engineers is crucial for developing and maintaining the AI and big data infrastructure. Robust data governance frameworks are essential for ensuring data quality, accuracy, and compliance with relevant regulations. A well-defined data governance framework Artikels clear roles and responsibilities, data quality standards, and procedures for handling data breaches. Finally, fostering a data-driven culture within the organization is critical for ensuring the successful adoption and utilization of AI and big data insights.
The Future of CRM
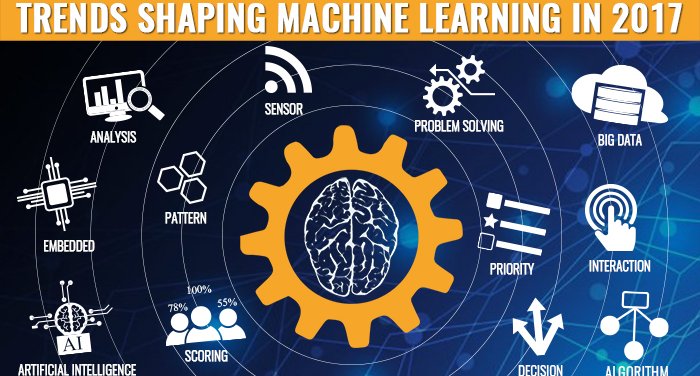
Source: dataconomy.com
The future of Customer Relationship Management (CRM) is inextricably linked to the continued advancement of artificial intelligence (AI) and big data analytics. We’re moving beyond simple data storage and retrieval towards a future where CRM systems proactively anticipate customer needs, personalize interactions with unprecedented accuracy, and automate complex processes with minimal human intervention. This evolution will reshape not only how businesses interact with their customers but also how they operate internally.
The convergence of AI and big data is fueling this transformation. AI algorithms, trained on massive datasets, enable CRM systems to perform predictive analytics, identify emerging trends, and automate tasks previously handled by human agents. This leads to increased efficiency, improved customer satisfaction, and ultimately, a significant boost to the bottom line. For example, AI-powered chatbots can handle routine customer inquiries 24/7, freeing up human agents to focus on more complex issues, while predictive models can anticipate customer churn and allow businesses to take proactive steps to retain valuable clients.
AI and Big Data’s Shaping of Future CRM
AI and big data will fundamentally alter the CRM landscape in several key ways. Predictive analytics will become even more sophisticated, enabling businesses to anticipate customer behavior with greater accuracy. This will allow for highly personalized marketing campaigns, targeted product recommendations, and proactive customer service interventions. Furthermore, AI-powered automation will streamline many CRM processes, reducing manual effort and improving efficiency. Imagine a CRM system that automatically segments customers based on their behavior, assigns leads to the most appropriate sales representatives, and even drafts personalized emails based on individual customer preferences – this is the future of CRM.
Impact of Emerging Technologies on CRM, CRM Trends to Watch: How Machine Learning and Big Data Are Shaping the Future
The Internet of Things (IoT), with its ability to collect vast amounts of data from connected devices, will significantly enrich CRM systems. Imagine a CRM that integrates data from smart home devices to understand customer preferences and tailor product offerings accordingly. Similarly, blockchain technology could enhance data security and transparency within CRM systems, particularly regarding customer data privacy and consent management. Blockchain’s immutable ledger could provide a verifiable record of customer interactions, ensuring data integrity and building trust. The integration of these technologies promises a more holistic and insightful view of the customer journey.
Evolution of CRM User Interfaces and User Experience
Future CRM systems will prioritize user experience (UX) to a far greater extent than current systems. Expect to see more intuitive interfaces, personalized dashboards, and seamless integration across multiple platforms. Voice-activated interfaces and augmented reality (AR) overlays are also likely to become more prevalent, enabling users to access CRM data and interact with the system in more natural and efficient ways. The goal is to create a CRM that is not just a tool, but an integral part of the workflow, seamlessly blending into the daily routines of sales, marketing, and customer service teams.
Timeline of Key Milestones in CRM Evolution
To illustrate the rapid pace of change, consider this simplified timeline:
Year | Milestone |
---|---|
1990s | Early CRM systems emerge, primarily focused on contact management and basic sales automation. |
2000s | Web-based CRM solutions become prevalent, improving accessibility and collaboration. |
2010s | Big data analytics and cloud computing significantly enhance CRM capabilities, enabling more sophisticated reporting and analysis. |
2020s – Present | AI and machine learning become integral to CRM, driving automation, personalization, and predictive analytics. Integration of IoT and blockchain technologies begins to reshape the CRM landscape. |
2030s and Beyond | Predictive CRM systems anticipate customer needs proactively, and hyper-personalization becomes the norm. Seamless integration across all business functions is achieved through AI-powered orchestration. |
Final Summary: CRM Trends To Watch: How Machine Learning And Big Data Are Shaping The Future
The future of CRM is undeniably intelligent, personalized, and proactive. By embracing machine learning, big data analytics, and AI-powered tools, businesses can unlock unprecedented levels of customer understanding and engagement. While challenges remain – particularly around data security and seamless integration – the rewards of a smarter, more efficient CRM system far outweigh the hurdles. The journey towards a truly data-driven CRM strategy is ongoing, but the destination – a world of hyper-personalized customer experiences – is undeniably worth the effort. So, buckle up and prepare for a CRM revolution.